CHANGE DETECTION IN ALUMINUM ELECTRODE IMAGE DURING OHMIC HEATING USING PRINCIPAL COMPONENT ANALYSIS
Abstract
In this paper, we propose a novel technique for unsupervised change detection dataset derived from a process pasteurization using aluminium plate left and right with frequencies 1kHz, 2kHz, 100Hz, 250Hz images using principal component analysis (PCA) and k-means clustering. The distinct image is partitioned into h × h non-overlapping blocks. orthonormal eigenvectors are extracted through PCA of non-overlapping block set to create an eigenvector space. Each pixel within the distinct image is characterized by a feature vector of a certain dimensionality. This feature vector is obtained projection the distinct image data onto the eigenvector space that has been generated. Change detection is accomplished by dividing the feature vector space into two clusters through the application of k-means clustering with k=2. Each pixel is then assigned to one of these two clusters based on the minimum Euclidean distance between the pixel's feature vector and the mean feature vector of the clusters. Empirical results validate the effectiveness of the proposed approach.
Downloads
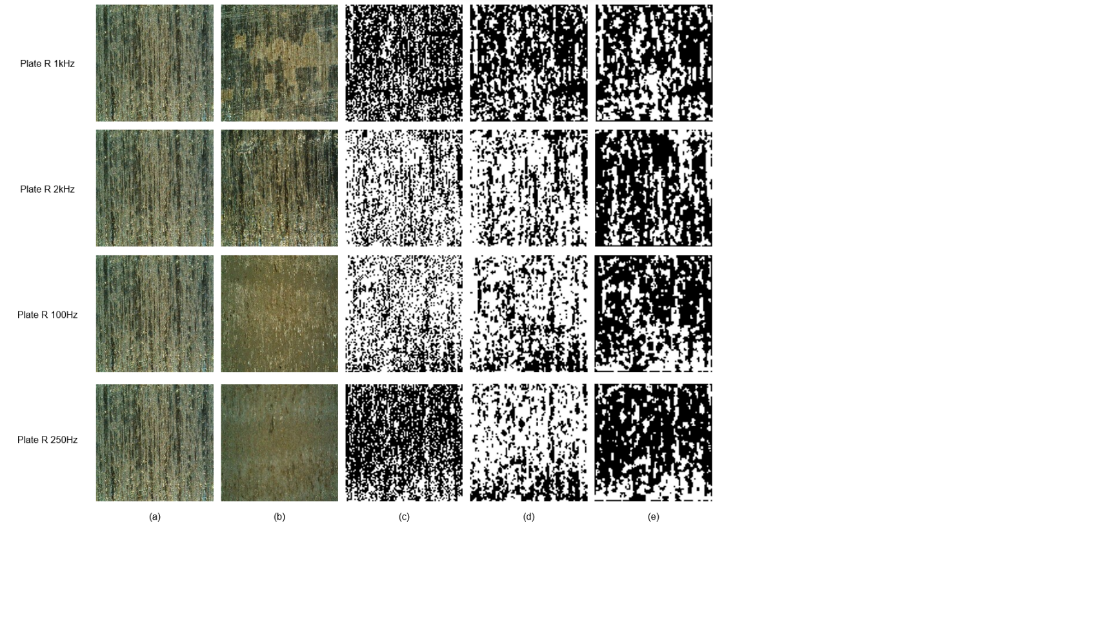
Copyright (c) 2024 Jurnal Mnemonic

This work is licensed under a Creative Commons Attribution-ShareAlike 4.0 International License.